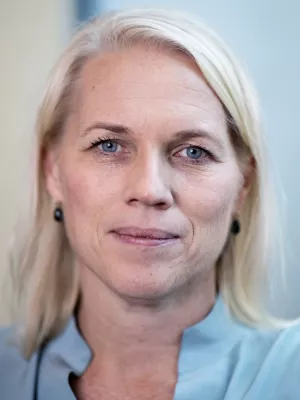
Sophia Zackrisson
Manager
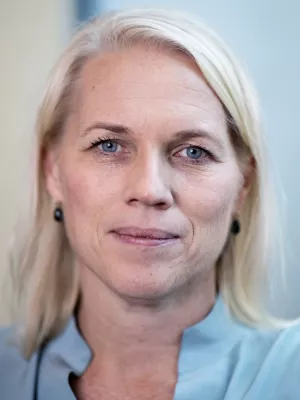
Can we reduce the workload of mammographic screening by automatic identification of normal exams with artificial intelligence? A feasibility study
Author
Summary, in English
Purpose: To study the feasibility of automatically identifying normal digital mammography (DM) exams with artificial intelligence (AI) to reduce the breast cancer screening reading workload. Methods and materials: A total of 2652 DM exams (653 cancer) and interpretations by 101 radiologists were gathered from nine previously performed multi-reader multi-case receiver operating characteristic (MRMC ROC) studies. An AI system was used to obtain a score between 1 and 10 for each exam, representing the likelihood of cancer present. Using all AI scores between 1 and 9 as possible thresholds, the exams were divided into groups of low- and high likelihood of cancer present. It was assumed that, under the pre-selection scenario, only the high-likelihood group would be read by radiologists, while all low-likelihood exams would be reported as normal. The area under the reader-averaged ROC curve (AUC) was calculated for the original evaluations and for the pre-selection scenarios and compared using a non-inferiority hypothesis. Results: Setting the low/high-likelihood threshold at an AI score of 5 (high likelihood > 5) results in a trade-off of approximately halving (− 47%) the workload to be read by radiologists while excluding 7% of true-positive exams. Using an AI score of 2 as threshold yields a workload reduction of 17% while only excluding 1% of true-positive exams. Pre-selection did not change the average AUC of radiologists (inferior 95% CI > − 0.05) for any threshold except at the extreme AI score of 9. Conclusion: It is possible to automatically pre-select exams using AI to significantly reduce the breast cancer screening reading workload. Key Points: • There is potential to use artificial intelligence to automatically reduce the breast cancer screening reading workload by excluding exams with a low likelihood of cancer. • The exclusion of exams with the lowest likelihood of cancer in screening might not change radiologists’ breast cancer detection performance. • When excluding exams with the lowest likelihood of cancer, the decrease in true-positive recalls would be balanced by a simultaneous reduction in false-positive recalls.
Department/s
- BioCARE: Biomarkers in Cancer Medicine improving Health Care, Education and Innovation
- Radiology Diagnostics, Malmö
Publishing year
2019-04-16
Language
English
Pages
4825-4832
Publication/Series
European Radiology
Volume
29
Issue
9
Document type
Journal article
Publisher
Springer
Topic
- Radiology, Nuclear Medicine and Medical Imaging
Keywords
- Artificial intelligence
- Breast cancer
- Deep learning
- Mammography
- Screening
Status
Published
Research group
- Radiology Diagnostics, Malmö
ISBN/ISSN/Other
- ISSN: 0938-7994