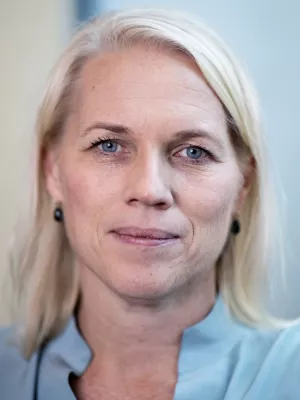
Sophia Zackrisson
Manager
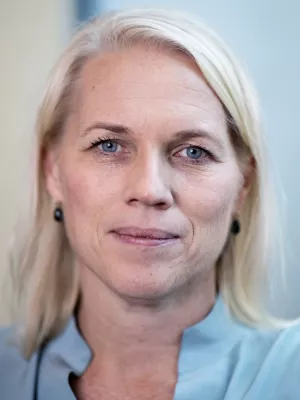
Artificial intelligence (AI) to enhance breast cancer screening : protocol for population-based cohort study of cancer detection
Author
Summary, in English
Introduction Artifi cial intelligence (AI) algorithms for interpreting mammograms have the potential to improve the effectiveness of population breast cancer screening programmes if they can detect cancers, including interval cancers, without contributing substantially to overdiagnosis. Studies suggesting that AI has comparable or greater accuracy than radiologists commonly employ € enriched' datasets in which cancer prevalence is higher than in population screening. Routine screening outcome metrics (cancer detection and recall rates) cannot be estimated from these datasets, and accuracy estimates may be subject to spectrum bias which limits generalisabilty to real-world screening. We aim to address these limitations by comparing the accuracy of AI and radiologists in a cohort of consecutive of women attending a real-world population breast cancer screening programme. Methods and analysis A retrospective, consecutive cohort of digital mammography screens from 109 000 distinct women was assembled from BreastScreen WA (BSWA), Western Australia's biennial population screening programme, from November 2016 to December 2017. The cohort includes 761 screen-detected and 235 interval cancers. Descriptive characteristics and results of radiologist double-reading will be extracted from BSWA outcomes data collection. Mammograms will be reinterpreted by a commercial AI algorithm (DeepHealth). AI accuracy will be compared with that of radiologist single-reading based on the di €erence in the area under the receiver operating characteristic curve. Cancer detection and recall rates for combined AI-radiologist reading will be estimated by pairing the first radiologist read per screen with the AI algorithm, and compared with estimates for radiologist double-reading. Ethics and dissemination This study has ethical approval from the Women and Newborn Health Service Ethics Committee (EC00350) and the Curtin University Human Research Ethics Committee (HRE2020-0316). Findings will be published in peer-reviewed journals and presented at national and international conferences. Results will also be disseminated to stakeholders in Australian breast cancer screening programmes and policy makers in population screening.
Department/s
- Radiology Diagnostics, Malmö
- LUCC: Lund University Cancer Centre
- EpiHealth: Epidemiology for Health
Publishing year
2022-01
Language
English
Publication/Series
BMJ Open
Volume
12
Issue
1
Document type
Journal article
Publisher
BMJ Publishing Group
Topic
- Cancer and Oncology
Keywords
- Breast imaging
- Breast tumours
- Diagnostic radiology
Status
Published
Research group
- Radiology Diagnostics, Malmö
ISBN/ISSN/Other
- ISSN: 2044-6055